6.6: Ecosystem Monitoring
( \newcommand{\kernel}{\mathrm{null}\,}\)
A complex and adaptive ecosystem in which all the chemical, physical, and biological components, functions, and processes are intact and functioning normally is considered a healthy ecosystem (but see Cumming and Cumming (2015), for a discussion on this value-based term). In contrast, disturbing any of an ecosystem’s components, functions, and/or processes will, by definition, alter them to some degree (Table 10.1). In many cases, ecosystems that have been exposed to certain forms and levels of disturbances remain healthy because there is redundancy in the roles performed by different ecosystem components (Section 4.2.1). This ability of an ecosystem to withstand certain forms and levels of disturbances is referred to as ecosystem stability. Ecosystem stability could be the result of one or both of two qualities: resistance and resilience. Resistance is the ability of an ecosystem to retain the same characteristic communities and natural cycles throughout and after a disturbance event, while resilience is the ability of an ecosystem to rapidly recover or adapt after a disturbance event. For example, if the number of native aquatic insect species decline after non-native fishes are introduced to previously fish-free ponds, the pond’s ecosystem has low resistance. But if the native insect community recovers rapidly after the non-native fishes were removed, the ecosystem is resilient.
Natural function |
Changes attributed to human activities |
---|---|
Land surface |
As much as half of the world’s ice-free land surface has been transformed to cater to people’s need for natural resources. Much of these changes are driven by agricultural activities. |
Nitrogen cycle |
Human activities release massive amounts of nitrogen into natural ecosystems on a daily basis. Much of this occurs through the use of nitrogen fertilisers, burning fossil fuels, and cultivating nitrogen-fixing crops. |
Atmospheric carbon cycle |
Scientists estimate that humans would have doubled levels of carbon dioxide in the Earth’s atmosphere by the middle of this century. This is primarily the result of fossil fuel use and deforestation. |
Wildlife populations |
Between 1970 and 2014, Sub-Saharan Africa have lost three quarters of its freshwater vertebrates; the rate of these declines shows no sign of reducing. |
Pollutants |
Pollution from human activities have become so omnipresent that it is hard to escape its impacts. Microplastics have been found in drinking water and the food we eat (Chapter 7). |
Sources: MEA, 2005; Kulkarni et al., 2008; http://www.livingplanetindex.org
Many forms of ecosystem disturbances are easy to observe. Consequently, monitoring these visible forms of disturbances—such as the outright destruction of a forest or plastic pollution on a beach—focuses less on detection and more on developing systematic survey protocols (Section 9.1) that can provide information on whether a disturbance is spreading and increasing in intensity, or whether conservation action is successful in containing the threat. However, some disturbances are subtler, unobtrusive, and thus difficult to detect; examples include pesticide drift and agricultural runoff (Section 7.1). Adopting a “wait and see” approach to detecting these invisible forms of disturbances can be particularly damaging, since that approach generally ends at a point where the harm will either be impossible to reverse or will require significantly more resources and time than would have been the case if the problem was addressed earlier. In this way, there are many similarities between monitoring ecosystem health and human health—some ailments are easier to diagnose than others, but we avoid the worst-case scenarios by screening regularly for diseases and treating the threatening ones promptly.
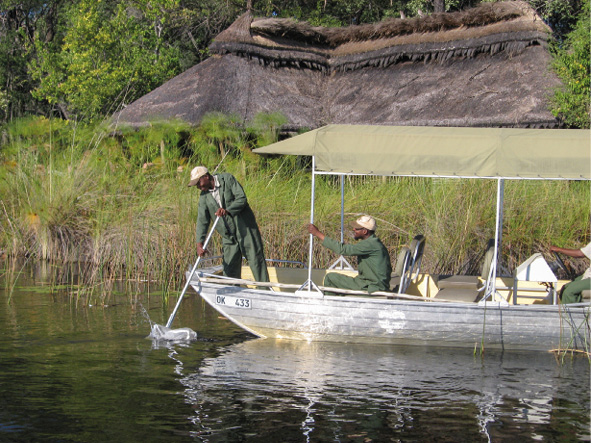
Perhaps the most popular method conservation biologists use to monitor ecosystem health is known as biomonitoring. By monitoring the abundance and/or fitness of sensitive species (Box 10.1), biologists can sometimes detect ecosystem degradation before it becomes apparent to the human eye or escalates to a point where it starts impacting human lives (Bornman and Bouwman, 2012). Monitoring environmental indicators such as macroinvertebrates (Figure 10.2) is particularly popular when examining the ecological condition of aquatic ecosystems; mayflies, caddisflies, and stoneflies—specialists of undisturbed streams—are often replaced by flies and midges in polluted and disturbed environments. Sometimes however, when plants or animals are not easily monitored, certain aspects of those species can still be monitored. One example is monitoring total plant biomass as a proxy for soil nutrients or intensity of herbivory. Another option is to perform a bioassay, during which a sensitive organism (typically water fleas or plankton) is released into a potentially contaminated environment to see if death or declining health occurs.
By monitoring the abundance and/or fitness of sensitive species, biologists can detect threats to biodiversity before it becomes apparent to the human eye.
Rosina Kyerematen
Department of Animal Biology and Conservation Science,
University of Ghana,
Legon, Ghana.
Insects are important to nearly every terrestrial food web in the world and serve a multitude of different purposes: some insects are responsible for pollination of plants while others are scavengers that clean up dead plant and animal material. In some cases, our understanding of an insect species’ ecological role can make it suitable as an indicator of environmental health. Biomonitoring looks at the presence and abundance of organisms within their natural communities to assess the impact of environmental disturbances; this knowledge can then be used to guide ecosystem management. An indicator taxon is one whose impact can be specifically and precisely measured; its abundance serves as a measure of the overall health of an ecosystem. Understanding how the presence and abundance of indicator species, and the relative abundance of tolerant and intolerant species, reflects the relative health of an environment can allow for rapid surveys of impaired ecosystems to assess trends as well as to track changes following remediation and restoration efforts.
Over the past few years, biomonitoring with insects as indicator taxa has become increasingly popular in Ghana. Butterflies are especially popular because they show varying relative sensitivities to environmental change; the abundance of certain butterfly species can for example be used to study the impact of habitat loss, fragmentation, and climate change (Kyerematen et al., 2018). The presence and abundance of butterflies more characteristic of open and disturbed ecosystems (Figure 10.A) can, for example, be used as an indicator of forest degradation.
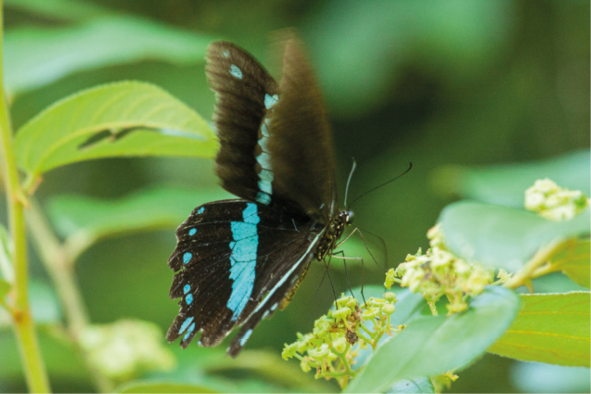
Aquatic insects, particularly benthic macroinvertebrates, are also useful bioindicators. Freshwater resources, such as lakes and rivers, provide water for drinking and washing to local people, and a home for economically important taxa, such as fish and shellfish. Protecting these water sources is therefore important for safeguarding people’s health and livelihoods. The presence, absence, and diversity of certain benthic macroinvertebrates, even at the order level, can provide valuable information about whether a waterbody is being degraded or not (Kyerematen et al., 2014; Nnoli et al., 2019). A recent study showed that dragonfly and damselfly diversities and populations along the coastal Densu River in Ghana vary widely depending on the physical condition of the river and surrounding area (Acquah-Lamptey et al., 2013).
With their high diversity and varying tolerances for ecosystem conditions, insects are extraordinarily suited as ecological indicators in environmental monitoring. Each insect species is also part of a wider biological community with important ecological roles. If lost, not only will an abundance of other life be affected, such a loss may also hint at a looming crisis facing people living in those compromised ecosystems.
At times, conservation biologists may need to measure the physical environment to assess environmental health. This approach is particularly common when tracking pollution, for example by monitoring for changes in biochemical indicators. For example, measuring total phosphorus, nitrogen, and dissolved oxygen load in streams and other surface water can help scientists track eutrophication (Section 7.1.1). Measuring these and other biochemical indicators is usually accomplished directly via chemical analysis of environmental samples, such as soil and water. Sometimes however, biochemicals indicators are tracked indirectly via biological samples obtained from plants and animals. Because they bio-accumulate heavy metals and other pollutants, filter feeders such as clams and mussels (e.g. Bodin et al., 2013) are particularly useful in this regard as they can be used to detect very low concentrations of harmful chemicals in the environment.
Monitoring ecosystems with geospatial analysis
A persistent challenge facing biologists who monitor ecosystems—and other aspects of biodiversity—is achieving consistency across space and time. Consider a survey of a sensitive bird community to track ecosystem change; not only will different observers have varying levels of experience, but they will almost certainly see different birds during an early morning census compared to one later in the afternoon due to differences in biology and behavior between species. These factors introduce error into monitoring data, which in turn can mask the effect a biologist tries to measure (Buckland and Johnston, 2017). Laboratory scientists’ control for these confounding factors by making multiple measurements under strictly controlled conditions. But for conservation biologists working outside in the wind and rain, repeated observations under similar conditions can be near impossible.
Geospatial analysis offers a variety of tools that allow biologists to overcome some of these traditional field monitoring challenges. These tools use geographic information systems (GIS) computer software packages to store, display, and manipulate a wide variety of data representing the natural environment, biodiversity, and human land-use patterns as they relate to one another on Earth’s surface. GIS thereby allows biologists to easily visualise and analyse spatial relationships between mapped data, which may include aspects such as vegetation types, climate, soils, topography, geology, water availability, species distributions, existing protected areas, human settlements, and human resource use (Figure 10.3). Understanding such relationships helps conservation biologists to prioritise their actions, for example by identifying areas where data are lacking, where an environmental change requires further investigation, or where gaps in regional protected areas network exist.
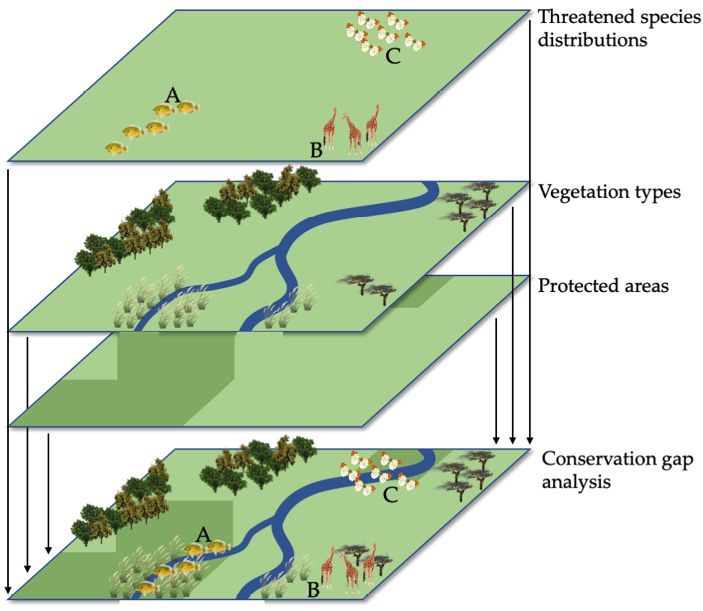
Remote sensing is a special branch of geospatial analysis directed at obtaining ecosystem data without making physical contact (i.e. boots on the ground) with the observation site. Before the turn of the 20th century, the most popular form of remote sensing was aerial photography from airplanes. These aerial photographs facilitated geographers’ ability to draw maps of landscape features, including human infrastructure and natural vegetation patterns. Remote sensing opportunities greatly expanded from 1960 onward, with the launch of the National Aeronautical Space Administration’s (NASA) first Earth observation satellites, to take photographs of Earth from space for weather forecasting. Subsequent satellite programs expanded their scope to also collect additional data of Earth’s surface and atmosphere. While much of this data would have been useful to conservation, early satellite data products were very expensive and thus largely out of reach of the larger conservation community. This all changed in 2008 when NASA started distributing their Earth observation products for free to the public, heralding an era in which remote sensing became a standard tool in the conservation field.
Remote sensing offers a variety of tools that allow biologists to monitor biodiversity beyond the abilities of traditional field monitoring techniques.
Today, hundreds of Earth observation satellites circle the planet, offering near real-time access to unbiased and consistent environmental datasets of nearly all terrestrial surfaces, oceanic surfaces and floor depths, and the atmosphere, all from the comfort of a computer connected to the internet (Wilson et al., 2013). Scientists use these products in ecosystem monitoring efforts, including monitoring water quality (Dube et al. 2015), forest loss (Laporte et al., 2007), coral reef health (McClanahan et al., 2011), desertification (Symeonakis et al., 2004), and fire regimes (Archbald et al., 2010). Linking the information obtained by Earth observation satellites to biological information collected on the ground has proved invaluable in monitoring species’ threat statuses (Di Marco et al., 2014), ecosystem connectivity (Wegmann et al., 2014), and habitat suitability (Torres et al., 2010), as well as understanding how biodiversity responds to environmental changes (Box 10.2).
Barend F. N. Erasmus
Global Change Institute (GCI), University of the Witwatersrand,
Johannesburg, South Africa.
Remote sensing is the art and science of observing objects or landscapes from a distance, without being in direct contact with the environment. Although you can think of wildlife photography as a type of remote sensing, the term usually refers to aerial photography (Figure 10.B), or images taken via satellite. For both cases, there is a trade-off between the area covered by each photo (the footprint), how much digital storage space is available, and how often a satellite takes a picture of the same area. For satellites that take pictures of the earth systematically, along a pre-defined path, cloud cover determines how often you can get a usable image. Recent improvements in technology, now allow for the deployment of constellations of satellites that have a collective point-and-track capability to observe an area almost continuosly.

Our eyes are sensitive to the colours red, green, and blue, together called the visible part of the electromagnetic spectrum. This is actually a very small part of the entire spectrum, and scientists have found, for example, that vegetation seen as uniformly green shows a lot of variation in the infrared part of the spectrum. For this reason, many satellites carry cameras that can “see” infrared light, and by proxy, measure vegetation health, biomass, and sometimes even structure. This capability, together with frequent revisit rates, allows for a unique view of how African landscapes change, whether through habitat loss, seasonal changes, or drought. Analyses of 13 years of remotely sensed data show that the most arid parts of southwest Botswana now experience typical summer vegetation conditions later in the year (Dubovyk et al., 2015), so herbivores must cope with a much longer dry season now than they experienced in 2000.
African landscapes are always changing, and sometimes in unpredictable ways—localised thunderstorms at the beginning of the rainy season can rapidly change a dry dustbowl landscape into green grazing. These “wet footprints” can cover areas as small as 1 km × 3 km; in contrast, a large frontal weather system can cover dozens or even hundreds of kilometres. An ungulate looking for green grazing not too far from drinking water, while trying to avoid predators and hunters, needs the ability to detect such green patches, and the strength and knowledge to move there. Obtaining this knowledge from an ungulate’s perspective of the landscape is no small task. Hopcraft et al. (2014) show how common wildebeest and plains zebra in the Serengeti have different migration strategies: wildebeest move to green grass as quickly as they can, with little effort to avoid predators, whereas zebra gauge predation risk and forage quality concurrent to their trek.
Our ability to understand ungulate movements and other ecosystem patterns has been greatly enhanced over the last few years, as animal-tracking technology (where GPS positions are logged and stored using radio-frequency tracking, mobile phone networks, satellite systems, or any combination) and the resulting analyses became more sophisticated. It is now possible to distinguish between locations where animals often spend a little time (for example, a preferred shady tree for resting during the heat of the day), or the same location where they infrequently spend a lot of time (for example, once during an oestrus cycle, a lactating lioness will spend a lot of time at her den, with cubs). It is also possible to “see” when such an animal changes “mode” of movement. For example, researchers have found that springbok (Antidorcas marsupialis, LC) rams around the Etosha pan in Namibia show sedentary behavior (small movements around a specific area) when grazing is good, which transitions to searching behavior during dry seasons (long, relatively fast movements in a straight line) as they move to areas that had water or grass during previous drought periods (Lyons et al., 2013).
If we combine this animal tracking capability with regular remotely sensed images of vegetation quality, then we can start to answer questions about why certain animals move to certain areas. It also highlights the fact that it is very seldom good enough to put a fence around an area and call it preserved. Due to the changing nature of Africa’s savannahs, animals need to be able to move to areas with water or forage, often outside reserves, when they become available. In the face of a changing climate and changing rainfall patterns, this ability to move long distance to reach vital resources remains one of the best adaptation responses that African ungulates may have to cope with climate change. However, fences, roads, and farmland may block these migrations across the landscape, putting those populations at risk of extinction (Section 5.1.1).
African conservation areas need to make provision for these animal movements, or risk conservation areas without sustainable animal populations. This presents a problem—how do we investigate options for large animals to move across a transformed rural landscape and minimise human-wildlife conflict while, on the other hand, still providing access to green grass or drinking water at a specific protected area? Both resources change in location and time of year, and only regular, detailed remote sensing of vegetation, combined with detailed animal movement studies, will provide the necessary picture in time and space.
The popularity and utility of these products have preceded and facilitated the expansion of other remote sensing applications in ecosystem monitoring. Among the most popular are radar products (Figure 10.4), which have become the standard method for obtaining elevation and other terrain data (NASA, 2009, 2013), as well as estimates of carbon stocks (Carreiras et al., 2012). Advances have also been made in using hyper-spectral imagery to monitor soil properties (Mashimbye et al., 2012) and even individual trees (Naidoo et al., 2012). LiDAR has enabled biologists to map three-dimensional vegetation, which can be used to explain animal movements (Loarie et al., 2013; Davies et al., 2016) and measure carbon stocks and forest loss (Burton et al., 2017).
Conservation biologists are often faced with the shifting baseline syndrome, where the reference points they use to measure their progress may be vastly different from earlier states.
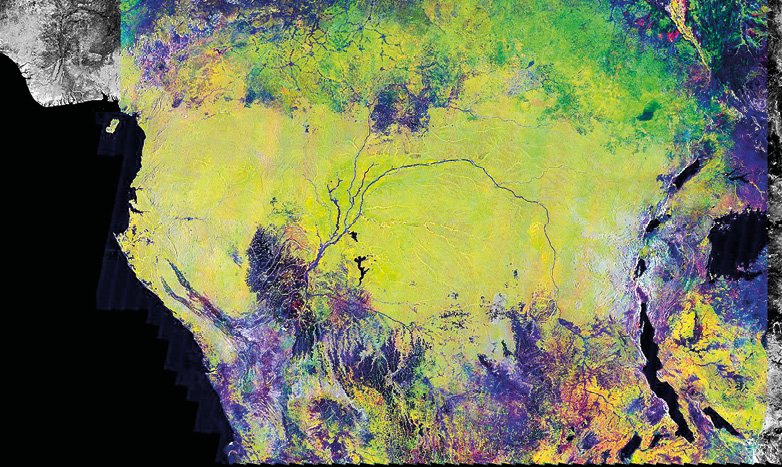
Despite the opportunities presented by remote sensing, it is critical to remember that it is not a substitute for traditional field monitoring methods. Most importantly, remote sensing applications cannot be considered reliable without verification using field data (see Burton et al., 2017). Most remote sensing products are also relatively new, which does not allow for enough opportunities to compare across time. In the absence of historical remotely sensed data, geospatial analysts usually choose the best reference site currently available; this may expose those analysts to shifting baseline syndrome, because the chosen reference site may be vastly different from earlier states the scientists are actually interested in studying (Bunce et al., 2008; Papworth et al., 2009). Remote sensing is, therefore, not a cure-all for ecosystem monitoring challenges; it is simply a powerful tool to supplement traditional field-based monitoring.